AI and Machine Learning: Today’s Implementation Realities Eğitimi
AI ve Makine Öğrenmesi: Günümüz Uygulama Gerçeklikleri Eğitimi, insanların artan bir hızla yapay zeka (AI) ve makine öğrenme (ML) teknolojilerini uygulamaya geçirmeleri için gereken bilgi ve becerileri kapsar.
Eğitim, AI ve ML kavramlarının temelini kuracak ve uygulama olarak kullanımının nasıl gerçekleştiğini açıklayacaktır. Eğitimde katılımcıları, AI ve ML algoritmalarının nasıl oluşturulduğunu, verilerin nasıl işlendiğini, verilerin nasıl modellere uygulandığını ve model sonuçlarının nasıl değerlendirildiğini öğrenecektir.
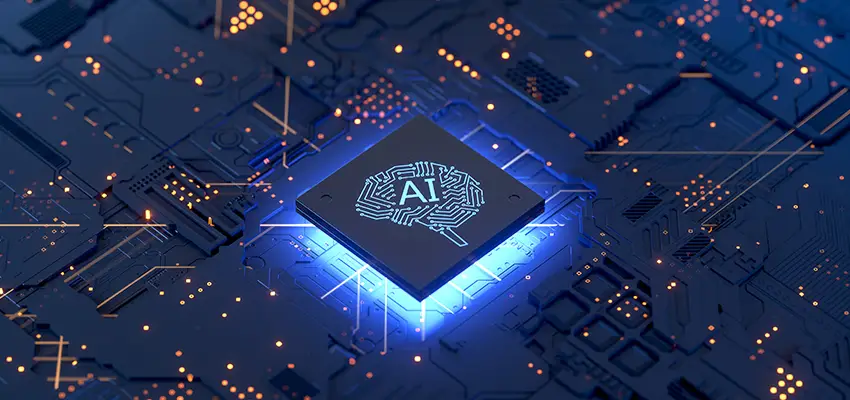
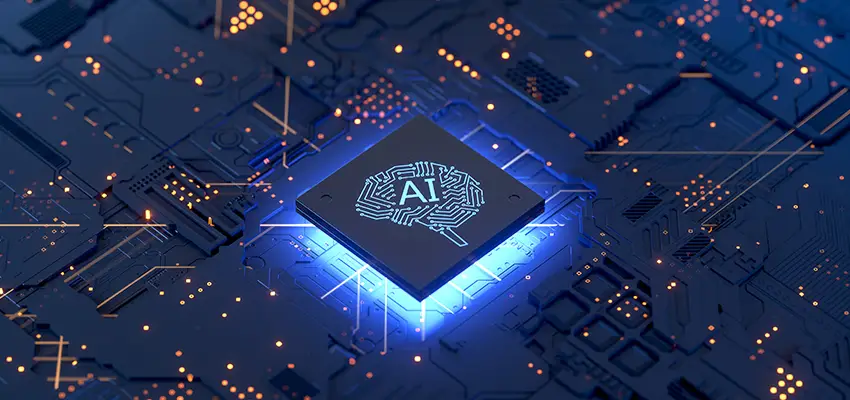
Eğitim Süresi: 3 Gün (18 Saat)
Eğitim İçeriği
What is AI, Machine learning and Data Science?
• What is the Organizational Value of AI & Machine Learning?
• How is Data Science Different from AI?
• Machine Learning
• What are the Skills Needed for Machine Learning?
• What Does a Data Scientist Do All Day?
Data Science Core Concepts
• Orientation to Big Data
• Trends within the analytically competitive organization
• The advent of Data Science
• What is machine learning’ role in Big Data?
• ROI of data science, big data and associated analytics
• The future of data science, big data and advanced analytics
How to Think Like A Data Scientist
• Stats 101 in ten minutes
• A / B testing and experiments
• BI vs predictive analytics
• IT’s role in predictive analytics
• Statistics and machine learning: complementary or competitive?
• Primary project types
• Common analytic and machine learning algorithms
• Popular tools to manage large-scale analytics complexity
• Performing a data reconnaissance
• Building the analytic sandbox
• Preparing train / test / validation data
• Defining data sufficiency and scope
The Cao’s Roadmap
• The Modeling Practice Framework™
• The elements of an organizational analytics assessment
• Project Definition: The blueprint for prescriptive analytics
• The critical combination: predictive insights & strategy
• Establishing a supportive culture for goal-driven analytics
• Defining performance metrics to evaluate the decision process
• What is the behavior that impacts performance?
• Do resources support stated objectives?
• Leverage what you already have
• Developing and approving the Modeling Plan
• Selecting the most strategic option
• Planning for deployment
• Measuring finalist models against established benchmarks
• Preparing a final Rollout Plan
• Monitoring model performance for residual benefit
Building The Goal-Centered Analytics Operation
• Attracting and hiring the right analytic talent
• The roles and functions of the fully-formed analytic project team
• Specialization in analytic project teams
• Analytic opportunity identification, qualification and prioritization
• Organizational resistance and developing a culture for change
• Project failure is not the worst outcome
• Staging the organizational mind shift to data-driven decisioning
• Motivating adoption by domain experts, end users and leadership
• Recording ongoing organizational changes
• Monitoring and advancing organizational analytic performance
• “Democratizing” analytics: Advantages and risks of “self-service”
• Standing up an agile analytic modeling factory
• Knowledge retention and skill reinforcement
• The Future of AI and Advanced Analytics
• From Rhetoric to Reality
• Biggest Driver of AI and Machine Learning Innovation
• What’s Next in Data Science, AI and Machine Learning?
• Defining Your Organization’s AI Reality
Bu eğitimi dilediğiniz zaman şirketinize/ekibinize özel olarak planlamak için bizimle iletişime geçin.
Eğitim ve danışmanlıklarımız hakkında bilgilendirmek için sizi arayalım
AI and Machine Learning: Today’s Implementation Realities Eğitimi
Kurumsal olarak planlayabileceğiniz bu eğitimle şirketiniz/takımınız çalışanlarının gerçek potansiyelini ortaya çıkarın. Bu eğitimin içeriğini ihtiyacınıza özel olarak düzenlemek için bizimle iletişime geçin.